
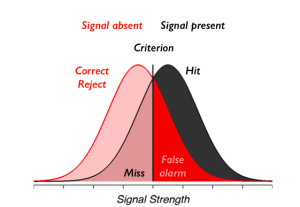
More complex stimuli are the norm, and to represent these the visual system must combine the outputs of the simpler mechanisms. Behaviorally, however, the stimuli that are ecologically relevant are not the simple features that these mechanisms are sensitive to. For example, simple cells are sensitive to luminance modulations of some specific frequency, orientation, and phase. The early visual system contains tuned mechanisms that respond to particular stimulus features at particular locations in the visual field.
#Signal detection theory explained software
The methods described here can be readily applied using software functions newly added to the Palamedes toolbox. We also show how one can fit the formulas directly to real psychometric functions using data from a binocular summation experiment, and show how one can obtain estimates of τ and test whether binocular summation conforms more to PS or AS. We show how the probability (and additive) summation formulas can be used to simulate psychometric functions, which when fitted with Weibull functions make signature predictions for how thresholds and psychometric function slopes vary as a function of τ, n, and Q. Both formulas are general purpose, calculating performance for forced-choice tasks with M alternatives, n stimuli, in Q monitored mechanisms, each subject to a non-linear transducer with exponent τ. We derive formulas that employ numerical integration to predict the proportion correct for detecting multiple stimuli assuming PS under SDT, for the situations in which stimuli are either equal or unequal in strength. Modeling the equivalent of PS under SDT is, however, relatively complicated, leading many investigators to use Monte Carlo simulations for the predictions. PS is traditionally modeled under high threshold theory (HTT) however, tests have shown that HTT is incorrect and that signal detection theory (SDT) is the better framework for modeling summation. There are broadly speaking two ways this can occur: additive summation (AS) where inputs from the different stimuli add together in a single mechanism, or probability summation (PS) where different stimuli are detected independently by separate mechanisms. A "utilized" signal detection theory offers the possibility of expanding the phenomena that can be understood within a decision-making framework.Many studies have investigated how multiple stimuli combine to reach threshold. This utility approach to signal detection theory clarifies otherwise enigmatic influences of perceptual uncertainty on measures of decision-making performance (accuracy and optimality) and on behavior (an inverse relationship between bias magnitude and sensitivity optimizes utility). In this tutorial, we show how incorporating the economic concept of utility allows signal detection theory to serve as a model of optimal decision making, going beyond its common use as an analytic method. Signal detection theory describes these types of decisions.
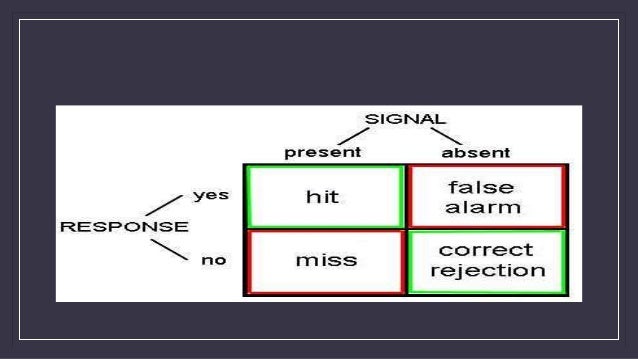
What do inferring what a person is thinking or feeling, judging a defendant's guilt, and navigating a dimly lit room have in common? They involve perceptual uncertainty (e.g., a scowling face might indicate anger or concentration, for which different responses are appropriate) and behavioral risk (e.g., a cost to making the wrong response).
